Facial recognition is a multifaceted problem. In our previous articles, we took an interest in the principles of facial recognition. Then we talked about the impact of COVID-19 on facial recognition AI, and more precisely the challenge for this technology to detect faces that are wearing masks.
But beyond the problems due to current constraints, we presented several algorithmic attacks to fool neural networks or infect their training database. But there are also other tricks to fool facial recognition. And these often use similar mechanisms to the one of wearing a mask.
What kind of attacks can AI be confronted with?
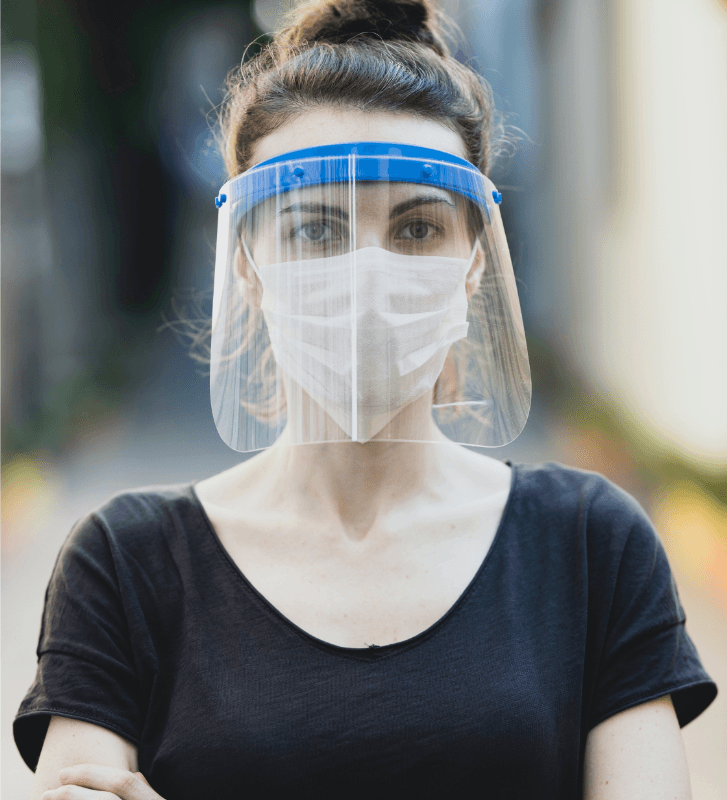
Attacks can fall into two categories: those that know the neural network structure to carry them out (white box), and those that work without knowing the structure (black box).
Currently, white box attacks are the most studied and the most numerous. Likewise, accessing training databases or neural network models to build these attacks is not always an easy task. Modifying all your training data (photos for example) so that you can no longer be identified is also not very practical to set up. Nevertheless, deceiving an image stream from a camera is another matter. Works have developed ways to hinder, or even mislead, facial recognition using physical objects that can be carried on one’s person. Indeed, either the system can no longer detect a person, or it is possible to pretend to be someone else. One of the almost elementary attacks on facial recognition systems is to use a simple photo to pretend to be someone else1. There are, of course, more sophisticated methods that will be less easy to counter.
What are the methods for fooling facial recognition algorithms?
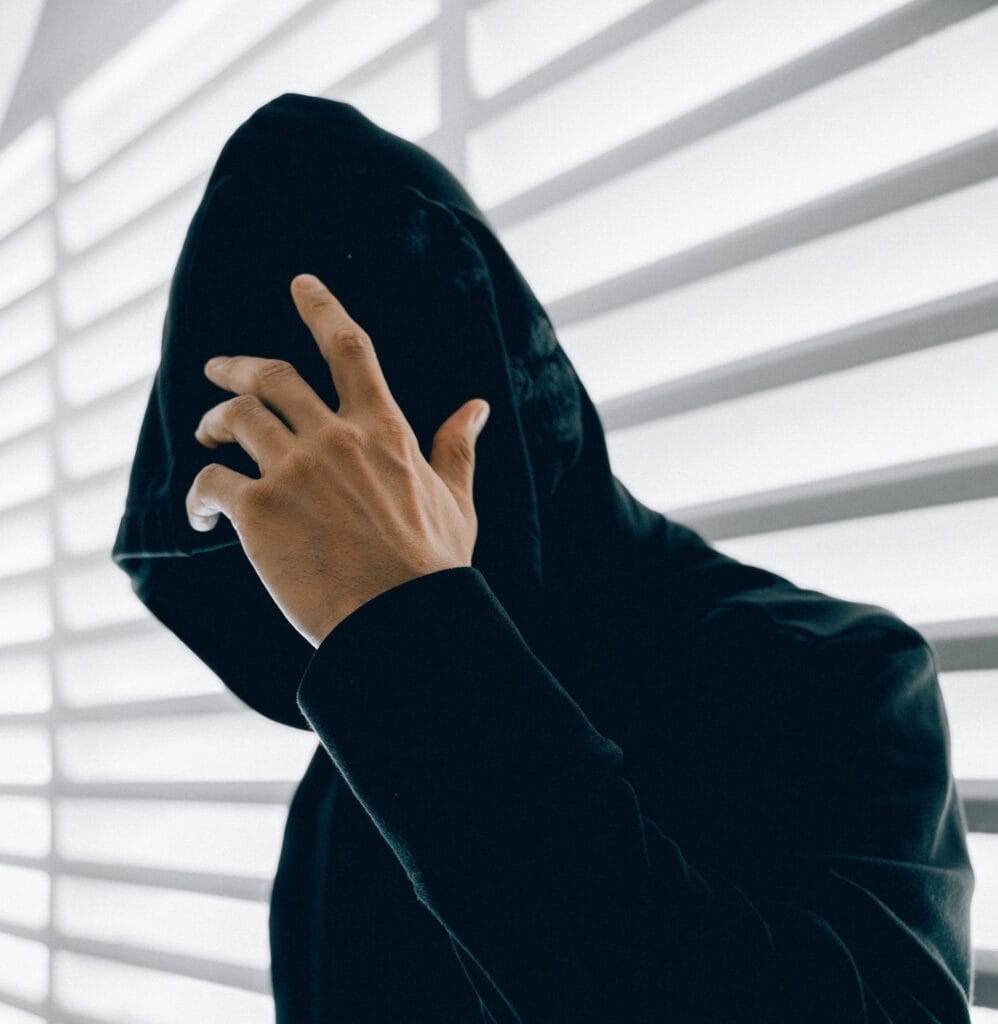
To begin, the American artist Adam Harvey has demonstrated that make-up, dyed wicks or stickers on the face are able to fool systems2. However, it is obvious that it is impossible to go unnoticed with this type of camouflage.
Other methods are using special glasses3. Either these allow the points of interest of the face to be blurred for the system, or they are equipped with quasi-infrared LEDs that will interfere with the camera’s vision without altering human vision. If the algorithm does not take the right points of interest, or if it is unable to recognize them because of a noise in the image (such as a halo of light), authentication will not be possible or will be erroneous.
Finally, clothing can also be used as a parry against recognition by camouflaging thermal signatures, or by blocking surrounding electromagnetic signals4. This type of clothing may become more widespread in the coming years, in response to the ever-increasing deployment of cameras.
To go further, high-precision 3D mask printing methods are possible. These masks can be used to fool algorithms that do not have enough reference points5. However, their realization is more complex and their efficiency questionable.
Other methods being tested
As in any Darwinian game of attack and defense, methods are being developed to counter these techniques6. Raphaël de Cormis, Vice President for Innovations and Digital Transformation at Thales, explains that the trend is to look for “proof of life”, i.e. elements that prove that what is detected is not inert. This can be based on skin movements/textures, detection of heartbeats, … The technique of molded masks could thus be ineffective. To do this, it is possible to ask the person to turn his head, blink his eyes… The systems then become difficult to deceive but new parries to counter this type of identification could quickly come into being. Notably based on Deep Fakes, allowing for example to artificially create a video that makes a face move by head movements, only from photos.
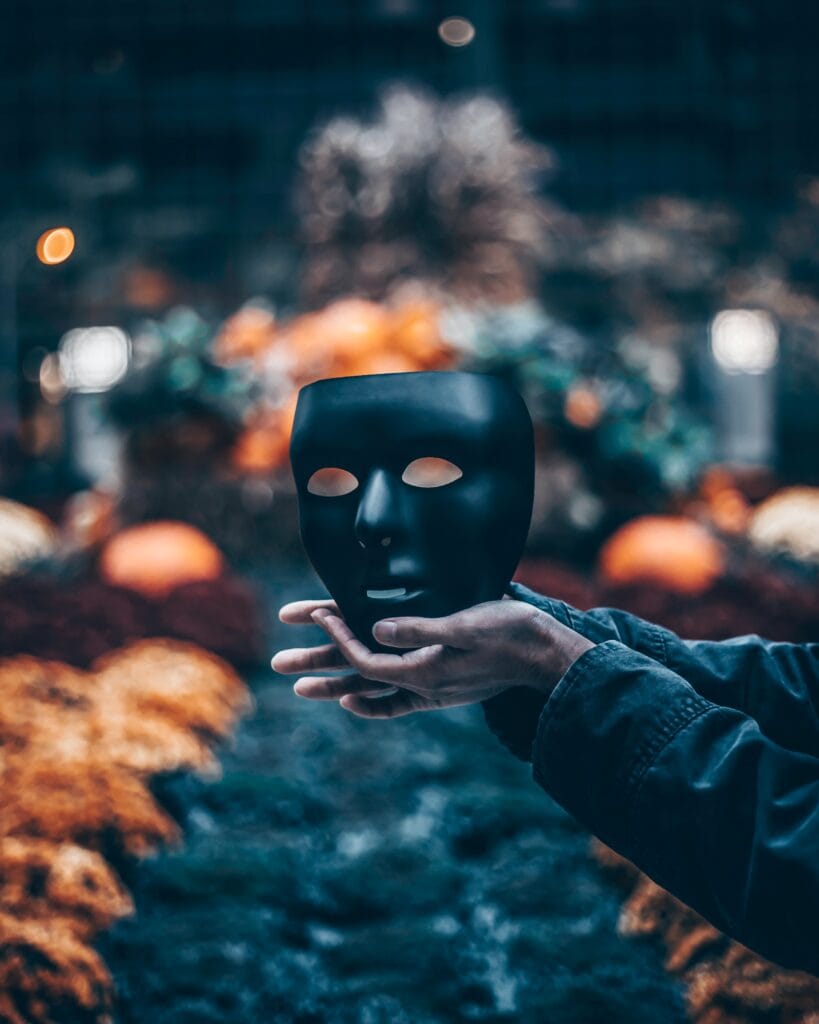
We notice that all these different techniques have one thing in common: they aim to camouflage the face to make it unrecognizable like a mask. The challenge, on the other hand, lies in the quality of these “artificial” masks. Will they be efficient enough to deceive the algorithms and thwart their security without being detected?
It is clear that systems will continue to improve and that it will always be more and more complicated to deceive them, but it will never be impossible. Cross-checking authentication methods {6} is a potentially very effective approach to improving system resiliency. Indeed, it allows the vulnerabilities offered by one authentication system to be compensated for by the strengths of another. It is then much more difficult to bypass several protection systems that are very different from each other. For example, facial recognition, coupled with biometric checks and code verification, would enable an extremely high level of security. What’s more, if one system fails, another can take over.
The development linked to the technological advances in facial recognition is quite long and above all it involves constantly improving systems to counter the Darwinian game of attacks/parries. The cross-referencing of authentication methods seems to be a way to reinforce security. It is not necessarily the most comfortable, but it allows everyone to be identified while limiting the risks.
Beyond the desire to deliberately mislead systems, they can also be subject to unintentional sources of error. Learning biases are a frequent source of error for artificial intelligence algorithms. We will address this topic in a future article!
- Des systèmes de reconnaissance faciale auraient été trompés par des masques et photos ↩︎
- Quand le design cherche à déjouer les logiciels de reconnaissance faciale | Radio-Canada ↩︎
- Des lunettes pour tromper la reconnaissance faciale ↩︎
- Comment certains échappent aux caméras de surveillance et à la reconnaissance faciale – CNET France ↩︎
- Des systèmes de reconnaissance faciale dupés par un masque 3D ↩︎
- Peut-on tromper la reconnaissance faciale ? | Les Echos ↩︎